CROSS- ATTENTIONAL IMAGE GEO-LOCALIZATION
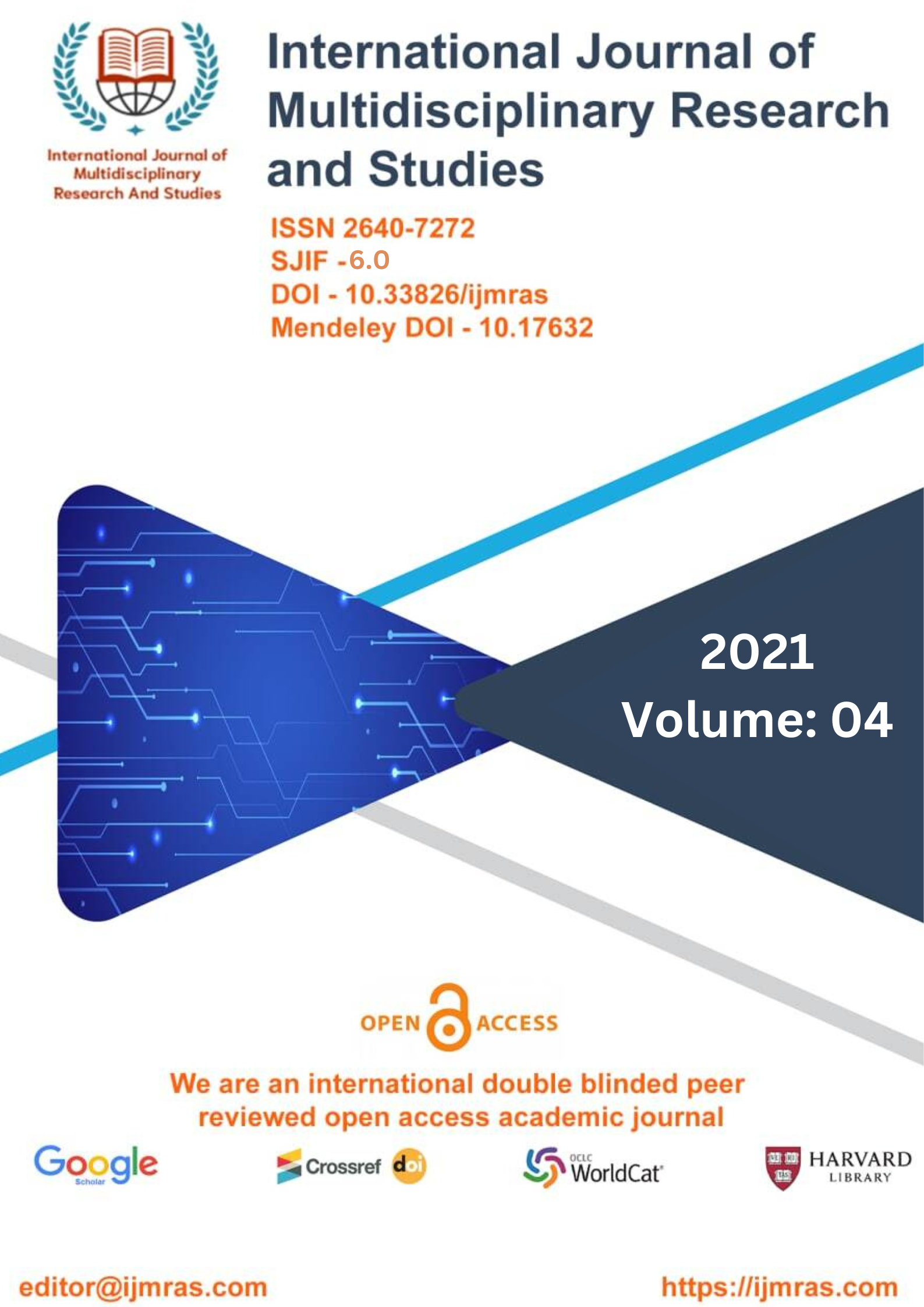
Abstract
Within the scope of this research, we investigate the problem of cross-view geo-localization, which attempts to establish the location of a street view image by comparing it to a collection of geo-tagged aerial pictures. Google is responsible for bringing this matter to our attention, and it will serve as the focus of this study. Cross-view matching is a particularly challenging operation to complete because of the large differences in look and geometry that exist between different views. We propose a novel evolving geo-localization Transformer (EgoTR) that models global dependencies by utilizing the qualities of self-attention in the Transformer. This allows us to better understand the relationships between different parts of the world. Using this strategy brings about a large reduction in the number of visual ambiguities that are present in the process of cross-view geo-localization. The currently available methods rely heavily on CNN. In addition, we make use of the positional encoding that Transformer provides in order to support the EgoTR in comprehending geometric arrangements between ground and aerial pictures and to correlate these configurations. EgoTR learns positional embeddings in a flexible manner via the training goal, in contrast to state-of-the-art approaches, which make stringent assumptions about the user's prior knowledge of geometry.
Keywords
Cross- Attentional, Image, stringent assumptions, geo-localization TransformerHow to Cite
References
Dave Pearson, Rethinking Image Sharing: Cleveland Trailblazers Link 20+ Hospitals and Provider Sites (2018).https://www.radiologybusiness.com/sponsored/1068/topics
/health-it/rethinking-image-sharing-cleveland-trailblazers-link-20-hospitals
MarcusDS, Olsen TR, Ramaratnam M, Buckner RL. The Extensible Neuro Imaging Archive Toolkit (XNAT): an informatics platform for managing, exploring, and sharing neuro imaging data. 2007 Spring;5(1):11-34.
Daniel K, Daniel R, Sandy N, Cesar R, and Chris B. “Managing Biomedical Image Metadata for Search and Retrieval of Similar Images". 2011 Aug.
Dina D-F, Sameer A, Mohammad-Reza S, Hamid S-Z, Farshad F, Kost E: Automatically Finding Images for Clinical Decision Support: IEEE Computer Society, 2007.
Hai J, et al: Content and semantic context-based image retrieval for medical image grid: IEEE Computer Society,2007
OriaV, etal: Modeling Images for Content-Based Queries: The DISIMA Approach, 1997
Solomon A, Richard C, Lionel B: Content-Based and Metadata Retrieval in Medical Image Database: IEEE Computer Society,2002
Warren R, et al. Mammo Grid—a prototype distributed mammographic database for Europe. Clin Radiol. 2007;62:1044–1051. doi: 10.1016/j.crad.2006.09.032.
Wesley WC, Chih-Cheng H, Alfonso FC, Cardenas AF, Ricky KT. Knowledge-Based Image Retrieval with Spatial and Temporal Constructs. IEEE Trans on Knowl and Data Eng. 1998;10:872–888. doi: 10.1109/69.738355.
Zhang GQ, Tao S, Xing G, Mozes J, Zonjy B, Lhatoo SD, Cui L. “N Hash: Randomized N-Gram Hashing for Distributed Generation of Val datable Unique Study Identifiers in Multicenter Research". JMIR Med Inform. 2015 Nov10;3(4): e35.
License
Copyright (c) 2021 Dipendra Kumar

This work is licensed under a Creative Commons Attribution 4.0 International License.
Individual articles are published Open Access under the Creative Commons Licence: CC-BY 4.0.