MULTI-CHANNEL ANALYSIS OF LONGITUDINAL DATA
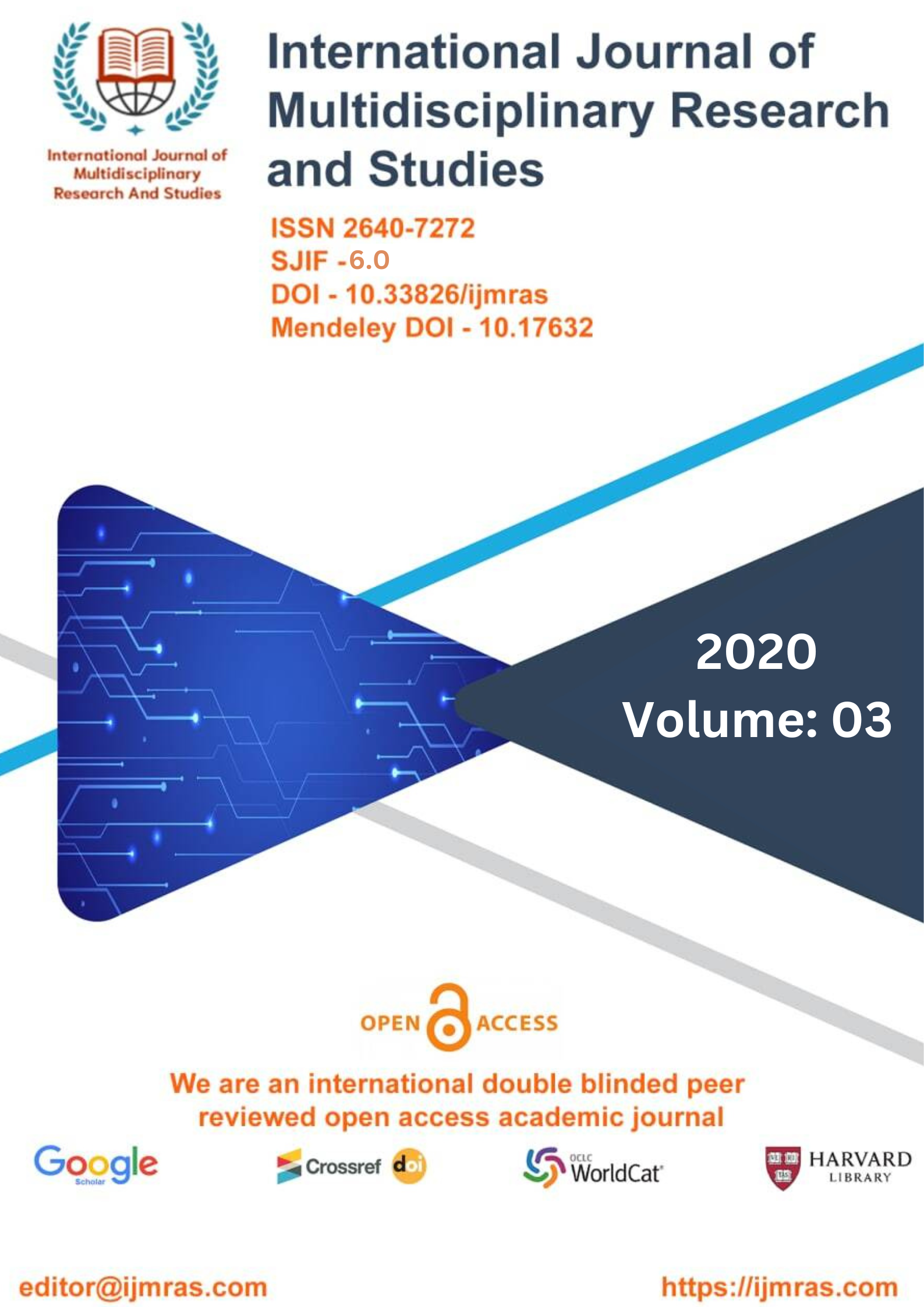
Abstract
However, comparing data from real-world health care settings is a difficult endeavor that offers some computational limitations, including excessive dimensionality, heterogeneity, temporal dependence, sparseness, and irregularity. In particular, healthcare-related data are typically collected over a wide range of resources, and the simultaneous evaluation of temporal correlations between multiple streams of data may arise from the dissemination of assets such as medicinal drugs. evaluation is required. diagnosis and methods. Underdeveloped nations are particularly prone to viral attacks due to the exceptionally contagious nature of the virus as well as sluggish growth in vaccination rates over the years. In recent years, approaches to nucleic acid identification have emerged as an essential factor in the method of glide screening of individuals. This trend is expected to continue. Reverse transcription polymerase chain reaction, sometimes called RT-PCR for its short form, is now the most accurate diagnostic tool available on the market.
Keywords
Multi-Channel, Longitudinal, RT-PCR, Accurate Diagnostic ToolHow to Cite
References
R. Mioto, F. Wang, S. Wang, X. Jiang, and JT Dudley, "Deep Learning for Healthcare: Review, Opportunities, and Challenges." Briefing in Bioinformatics, 1–11″ 2017.
S. Hochreiter and J. Schmidhuber, "Long Short Term Memory," Neural Comput., Vol. 9, pp. 1735–1780, November 1997.
Graves, "Generating Sequences with Recurrent Neural Networks," ArXiv e-print, August 2013.
F. Ma, R. Chitta, J. Zhou, Q. Yu, T. Sun, and J. Gao, "DiPol: Diagnosis Prediction in Healthcare Via Attention-Based Bidirectional Recurrent Neural Networks," Proceedings of the 23rd ACM SIGKDD International Conference on Knowledge Discovery and Data Mining, pp. 1903–1911, 2017.
T. Luong, H. Pham, and C. D. Manning, "Effective Approach to Attention-Based Neural Machine Translation," in Proceedings of the 2015 Conference on Empirical Methods in Natural Language Processing, (Lisbon, Portugal),
1412–1421, Association for Computational Linguistics, 2015.
D. Bahdanau, K. Chow, and Y. Bengio, "Neural machine translation by joint learning to align and translate," arXiv preprint arXiv:1409.0473, 2014.
a. Vaswani, N. Shazier, N. Parmar, J. Uszkorit, L. Jones, Ann Gomez, L. You. Kaiser, and I. Polosukhin, "Attention is all you need," Advances in Neural Information Processing Systems 30 (I. Guyon, UV Luxberg, S. Bengio, H. Wallach, R. Fergus, S. Viswanathan, and R. Garnett, ed.), pp. 5998–6008, Curran Associates, Inc., 2017.
E. Choi, MT Bahadori, A. Schuetz, WF Stewart, and J. Sun, "Doctor AI: Prediction of clinical events via recurrent neural networks," in Machine Learning for Healthcare Conference, pp. 301–318, 2016.
E. Choi, MT Bahadori, JA Kulas, A. Schuetz, WF Stewart, and J. Sun, "Retain An Interoperable Predictive Model for Healthcare Using Reverse Time Attention Mechanism," arXiv Preprint arXiv:1608.05745, 2016.
T. Pham, T. Tran, D. Phung, and S. Venkatesh, "DeepCare: A deep dynamic memory model for predictive medicine," in Pacific-Asia Conference on Knowledge-Edge Discovery and Data Mining, pp. 30–41, Springer, 2016.
In Proceedings of the AAAI Conference E. Choi, Z. Joo, Y. Lee, M. Dusenberry, G. Flores, E. Zu, and A. Dai, "Learning the Graphical Structure of Electronic Health Records with Graphical Transformers", On Artificial Intelligence, vol. 34, pp. 606–613, 2020.
H. Song, D. Rajan, J. Thiagarajan, and A. Espanias, "Attend and Diagnosis: Clinical Time Series Analysis Using Attention Models," Proceedings of the AAAI Conference on Artificial Intelligence, vol. 32, 2018.
why. Wang, X. Xu, T. Jin, X. Lee, G. Xie, and J. Wang, "Inpatient2VC: Medical Representation Learning for Inpatients," 2019 IEEE International Conference on Bioinformatics and Biomedicine (BIBM), pp. 1113–1117, IEEE, 2019.
J. Shang, T. Ma, c. Jiao and J. Sun, "Pre-training of graph augmented transform-formers for drug recommendation," arXiv preprint arXiv:1906.00346, 2019.
License
Copyright (c) 2020 Santosh kumar kaushal

This work is licensed under a Creative Commons Attribution 4.0 International License.
Individual articles are published Open Access under the Creative Commons Licence: CC-BY 4.0.