APPLICATION OF MACHINE LEARNING WITH A REFERENCE TO ELECTRICITY THEFT DETECTION
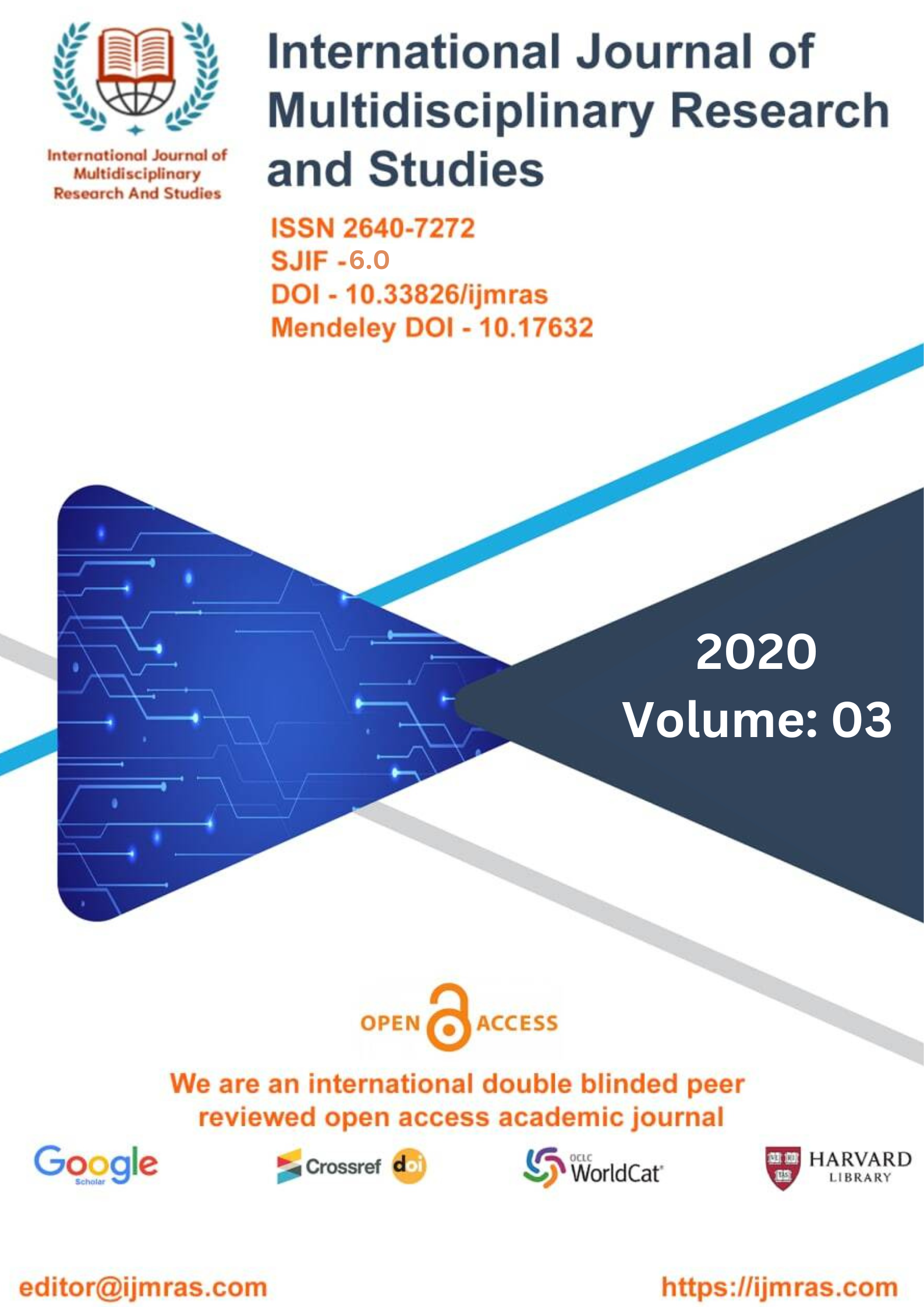
Abstract
That 's important to keep in mind as you go through their pictures. We agree with those observations and propose a change to the six robbery scenarios that would be additional as it should be with reporting close to real-international robbery styles and then practicing them in datasets aimed at realistic assessment and numerical evaluation. We're now using an algorithm that utilizes gadget mastering that will spot the robbery. Checking the power usage pattern of a consumer for irregularities is one way of detecting pilferage by that consumer. An easier way is to evaluate a person's behavior when starting with essential information about the person. We set up a supervised gadget study-based fully theft detection version with the goal of determining whether an unusual or fraudulent use pattern has taken place in a smart grid (SG) meter. For non-technical loss (NTL) detection, we rely on the advantage of XGBoost, a gradient boosting classifier (GBC), over the algorithm that studies discrete devices.
Keywords
Machine Learning, Electricity, robbery styles, detecting pilferageHow to Cite
References
C Andrews. [online]. Available: http://creativecommons.org/licenses/by-sa/3.0
H. T. , Bei Zhang, Y., "Big Data Analytics in Smart Grids: A Review," Energy Information, Vol. 1, pp. 3–4, 2018. [Online]. Available: https://energyinformatics.springeropen. com/articles/10.1186/s42162-018-0007-5
Xantacross. [online]. Available: https://commons.wikimedia.org/wiki/File:Euclidean_vs_DTW.jpg
A. Mamer and K. Benhamad, "Machine learning techniques for energy theft detection in AMI." New York, NY, USA: Computing Machinery Association, 2018. [online]. Available: https://doi.org/10.1145/3178461.3178484
P.-N. Tan, M. Steinbach, and V. Kumar, Introduction to Data Mining. Addison Wesley, May 2005.
KMU Ghori, M. Imran, A. Nawaz, RA Abbasi, A. Ullah, and L. Sajathmari, "Performance Analysis of a Machine Learning Classifier for Non-Technical Loss Detection," J Ambient Intl Human Comput, 2020.
I. Breedo, V. Kaverin, D. Abisheva, and A. Kolychev, "Monitoring of leakage currents of suspension insulators of high voltage overhead power lines."
J. Parmar, "Total Losses in Power Distribution and Transmission Lines: EEP," December 2017. [online]. Available at: https://electrical-engineering-portal.com/ Total-losses-in-power-distribution-and-transmission-lines-1
J. Navani, N. Sharma, and S. Sapra, "Technical and Non-Technical Losses in Power System and Its Economic," 2012.
S. Amin, GA Schwartz, AA Cardenas, and SS Shastri, "Game-theoretic model of power theft detection in smart utility networks: Providing new capabilities with advanced metering infrastructure," IEEE Control Systems Magazine, vol. 35, no. 1, pp. 66–81, 2015.
Northeast Group, LLC, "Power Theft and Nontechnical Losses: Global Markets, Solutions and Vendors," 2017.
L. Arango, E. Deccache, B. Bonatto, H. Arango, and E. Pamplona, "Study of the impact of electricity theft on the economy of a regulated electricity company," Journal of Control, Automation and Electrical Systems, vol. 28, June 2017.
F. Jameel and E. Ahmed, "An Empirical Study of Electricity Theft from Electricity Distribution Companies in Pakistan," Pakistan Development Review, Vol. 53, no. 3, pp. 239–254, 2014.
T. Smith, "Electricity Theft: A Comparative Analysis," Energy Policy, Vol. 32, pp. 2067–2076, February 2004.
P. Conceicao, "Human Development Report (2019): Beyond income, beyond average, beyond today: Inequalities in human development in the 21st century," 2019.
License
Copyright (c) 2020 Chanchala Kumari

This work is licensed under a Creative Commons Attribution 4.0 International License.
Individual articles are published Open Access under the Creative Commons Licence: CC-BY 4.0.