SYSTEM BASED ON NMFS PREDICTING RELATIONSHIP BETWEEN ENTITIES IN BIOMEDICAL DATABASE
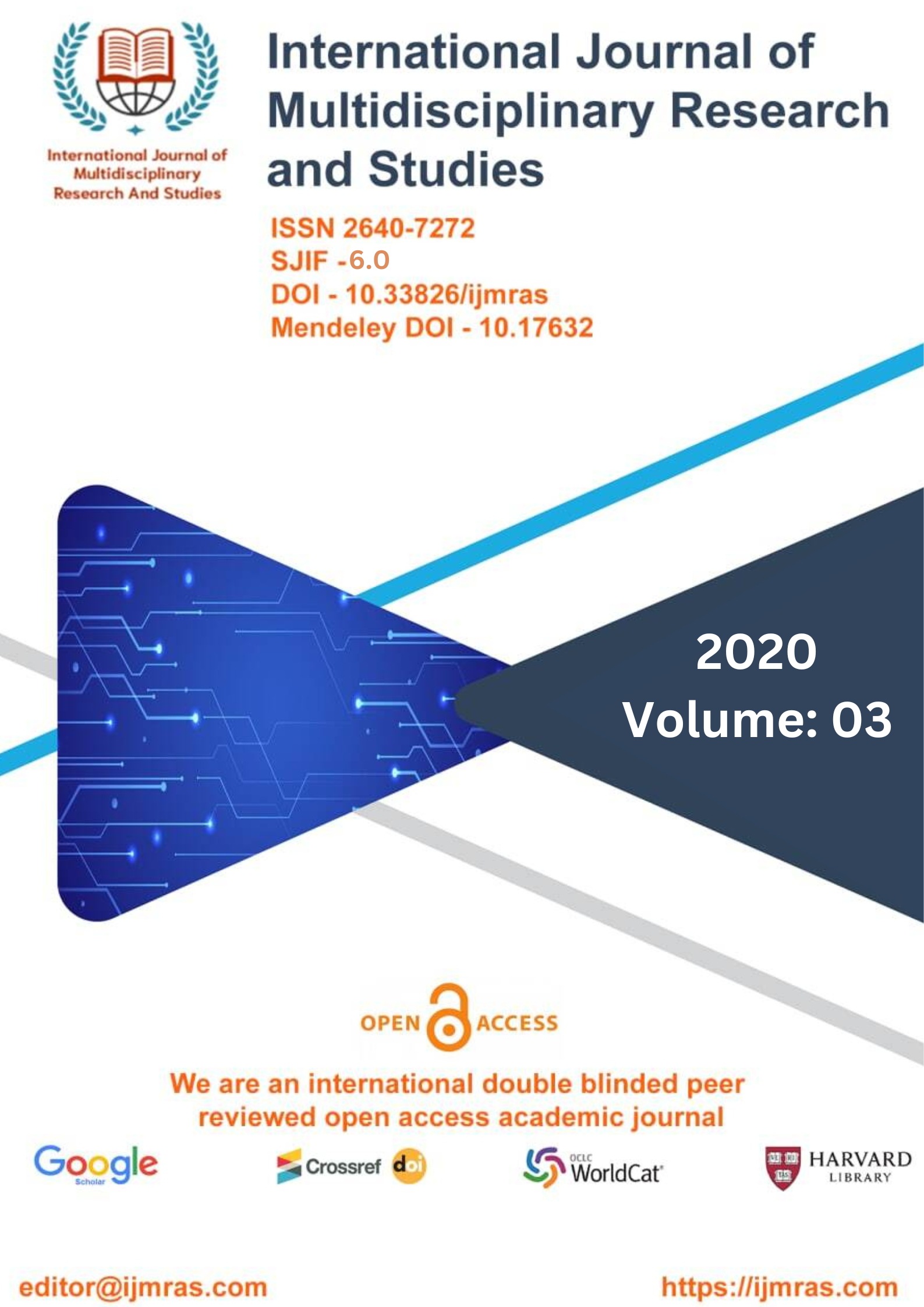
Abstract
Recommender systems are widely used to provide users with recommendations based on their preferences. With the ever-growing volume of information online, recommender systems have been a useful tool to overcome information overload. The utilization of recommender systems cannot be overstated, given its potential influence to ameliorate many over-choice challenges. There are many types of recommendation systems with different methodologies and concepts. Various applications have adopted recommendation systems, including e-commerce, healthcare, transportation, agriculture, and media. This paper provides the current landscape of recommender systems research and identifies directions in the field in various applications. This article provides an overview of the current state of the art in recommendation systems, their types, challenges, limitations, and business adoptions. To assess the quality of a recommendation system, qualitative evaluation metrics are discussed in the paper.
Keywords
NMFS, PREDICTING, BIOMEDICALHow to Cite
References
Kumar, Rajesh, and Zhang, Xiaosong and Wang, Wen and Khan, Riaz and Kumar, Jai and Sharif, Abubakar. (2019). A multimodal malware detection technique for Android IoT devices using multiple features. IEEE Access. pp. 1-1. 10.1109/access.2019.2916886.
Yadav, Chandra and Kumar, Rahul and Yadav, Aruna and Patnaik, Himanshu and Kumar, Ravindra and Khan, Arfat Ahmed and Haque, Mohammad Anul and Alhusen, Ahmed and Alharbi, Sultan. (2022). Malware analysis in IoT and Android systems with defensive mechanisms. Electronics. 11. 2354. 10.3390/Electronics11152354.
Ren, Zhongru& Wu, Haomin&Ning, Qian&Hussain, Iftekhar& Chen, Bingcai. (2020). End-to-end malware detection for Android IoT devices using Deep Learning. ad hoc network. 101. 102098. 10.1016/j.adhoc.2020.102098.
Kumar, Rajesh and Wang, Wenyoung and Kumar, Jai and Jamali, Zakaria and Yang, Ting and Ali, Waqar and Sharif, Abubakar. (2021). IoTMalware: Android IoT malware detection based on Deep Neural Network and Blockchain technology.
B, Haritha and Rajendran T,. (2022). Improved malware detection for IoT devices using Random Forest algorithm as compared to Decision Tree algorithm. 10.3233/APC220085.
Odusami, Modupe and Abayomi-Alli, Olusola and Mishra, Sanjay and Shobayo, Olamilekan and Damasevichius, Robertus and Maskeliunas, Ritis. (2018). Android Malware Detection: A Survey. 10.1007/978-3-030-01535-0_19.
Vinod, P.. (2014). Towards Android malware detection using ensemble features. 9. 375–387.
Ham, Hyo-sik and Kim, Hwan-hee and Kim, Myung-sup and Choi, Mi-jung. (2014). Linear SVM-based Android malware detection for reliable IoT services. Journal of Applied Mathematics. 2014. 1–10. 10.1155/2014/594501.
Liu, Xiaoli and Du, Xiaojiang and Zhang, Xiaosong and Zhu, Qingxin and Wang, Xie and Guizani, Mohsen. (2019). Adverse Samples on Android Malware Detection System for IoT Systems. sensor. 19. 974. 10.3390/s19040974.
Mathur, Akshay, and Podila, Lakshmi and Kulkarni, Keyur and Niaz, Qamar and Javed, Ahmed. (2021). NATICUSdroid: A malware detection framework for Android using native and custom permissions. Journal of Information Security and Applications. 58. 102696. 10.1016/j.jisa.2020.102696.
Omar, Maria and Zeebri, Subhi and M. Sadiq, Mohammad and Salim, Bara and Mohsin, Sana and Najat, Zareen and Haji, Laylan. (2021). Efficiency of Malware Detection in Android Systems: A Survey. Asian Journal of Computer Science and Information Technology. 7. 59-69. 10.9734/ajrcos/2021/v7i430189.
Kanteti, Uday and McMillin, Bruce. (2017). Multiple protection domain model of a vehicle in an automated platoon. 81–97. 10.1007/978-3-319-70395-4_5.
Chattopadhyay, Anupam, and Lam, Kwok-yan and Tavva, Yashwant. (2020). Autonomous Vehicles: Safety by Design. IEEE Transactions on Intelligent Transport Systems. pp. 1-15. 10.1109/tits.2020.3000797.
Jahan, Farah and Son, Viking and Niaz, Qamar and Alam, Mansoor. (2019). Security modeling of autonomous systems: A survey. ACM Computing Survey. 52. 1-34. 10.1145/3337791.
Hao, Jingjing and Han, Guangsheng. (2020). On the modeling of automotive safety: A survey of methods and perspectives. Internet of the future. 12. 198. 10.3390/ fi12110198.
Chattopadhyay, Anupam and Lam, Kwok-yan. (2018). Autonomous Vehicles: Safety by Design.
Hamd, Mohd. (2020). A multilayer secure framework for vehicle systems.
Ryan, Jamal. (2018). Data and cyber security in autonomous vehicle networks. Journal of Transportation and Telecommunications. 19. 325–334. 10.2478/TTJ-2018-0027.
Bouchelaghem, Siham and Bouabdallah, Abdelmadjid and Omar, Mawloud. (2021). Autonomous vehicle security: a literature review of real attack experiments. 10.1007/978-3-030-68887-5_15.
Le, Tuan, Lu, you and Gerla, Mario. (2015). Social caching and content retrieval in disruption tolerant networks (DTNs). 10.13140/2.1.4204.8009.
Gao, Wei and Cao, Guohong and Iyengar, Arun and Srivatsa, Mudhakar. (2014). Cooperative caching for efficient data access in interruption-tolerant networks. Mobile Computing, IEEE Transactions on. 13. 611–625. 10.1109/TMC.2013.33.
License
Copyright (c) 2020 Brajkishore Pandit

This work is licensed under a Creative Commons Attribution 4.0 International License.
Individual articles are published Open Access under the Creative Commons Licence: CC-BY 4.0.